E-Books →The Handbook of NLP with Gensim Leverage topic modeling to uncover hidden patterns, themes
Published by: voska89 on 21-03-2024, 00:45 | 0
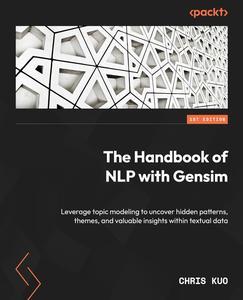
Free Download The Handbook of NLP with Gensim: Leverage topic modeling to uncover hidden patterns, themes, and valuable insights within textual data by Chris Kuo
English | October 27, 2023 | ISBN: 1803244941 | 310 pages | PDF | 23 Mb
Elevate your natural language processing skills with Gensim and become proficient in handling a wide range of NLP tasks and projectsKey FeaturesAdvance your NLP skills with this comprehensive guide covering detailed explanations and code practicesBuild real-world topical modeling pipelines and fine-tune hyperparameters to deliver optimal resultsAdhere to the real-world industrial applications of topic modeling in medical, legal, and other fieldsPurchase of the print or Kindle book includes a free PDF eBookBook Description
Navigating the terrain of NLP research and applying it practically can be a formidable task made easy with The Handbook of NLP with Gensim. This book demystifies NLP and equips you with hands-on strategies spanning healthcare, e-commerce, finance, and more to enable you to leverage Gensim in real-world scenarios.
You'll begin by exploring motives and techniques for extracting text information like bag-of-words, TF-IDF, and word embeddings. This book will then guide you on topic modeling using methods such as Latent Semantic Analysis (LSA) for dimensionality reduction and discovering latent semantic relationships in text data, Latent Dirichlet Allocation (LDA) for probabilistic topic modeling, and Ensemble LDA to enhance topic modeling stability and accuracy.
Next, you'll learn text summarization techniques with Word2Vec and Doc2Vec to build the modeling pipeline and optimize models using hyperparameters. As you get acquainted with practical applications in various industries, this book will inspire you to design innovative projects. Alongside topic modeling, you'll also explore named entity handling and NER tools, modeling procedures, and tools for effective topic modeling applications.
By the end of this book, you'll have mastered the techniques essential to create applications with Gensim and integrate NLP into your business processes.What you will learnConvert text into numerical values such as bag-of-word, TF-IDF, and word embeddingUse various NLP techniques with Gensim, including Word2Vec, Doc2Vec, LSA, FastText, LDA, and Ensemble LDABuild topical modeling pipelines and visualize the results of topic modelsImplement text summarization for legal, clinical, or other documentsApply core NLP techniques in healthcare, finance, and e-commerceCreate efficient chatbots by harnessing Gensim's NLP capabilitiesWho this book is for
This book is for data scientists and professionals who want to become proficient in topic modeling with Gensim. NLP practitioners can use this book as a code reference, while students or those considering a career transition will find this a valuable resource for advancing in the field of NLP. This book contains real-world applications for biomedical, healthcare, legal, and operations, making it a helpful guide for project managers designing their own topic modeling applications.Table of ContentsIntroduction to NLPWord EmbeddingText Wrangling and PreprocessingLatent Semantic Analysis with scikit-learnCosine SimilarityLatent Semantic Indexing with GensimUsing Word2VecDoc2Vec with GensimUnderstanding Discrete DistributionsLatent Dirichlet AllocationLDA ModelingLDA VisualizationThe Ensemble LDA for Model StabilityLDA and BERTopicReal-World Use Cases
Buy Premium From My Links To Get Resumable Support,Max Speed & Support Me
Links are Interchangeable - Single Extraction
Related News
-
{related-news}